はじめに
「IP-Adapter-FaceID-PlusV2」を使うと1枚の顔写真からその人物の写真を何枚も作成することが可能になります。touch-sp.hatenablog.com
顔の再現性は完璧ではありません。
しかし、作成する画像の一貫性は高いです。
実例を見た方がわかりやすです
元画像
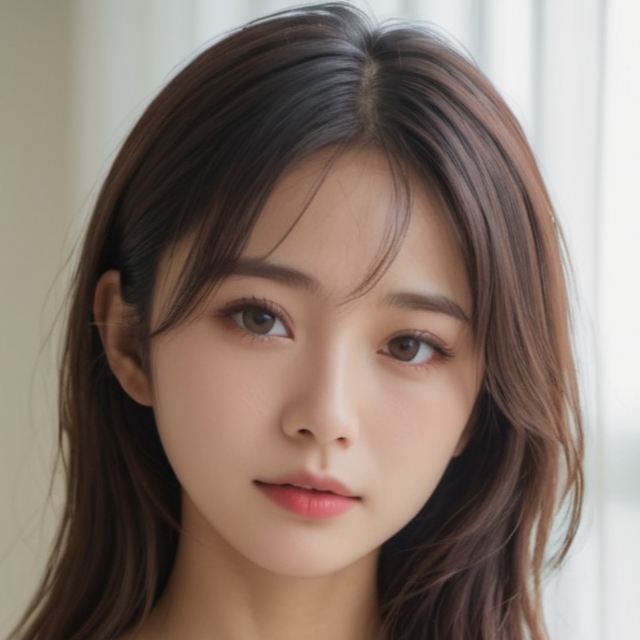
作成画像
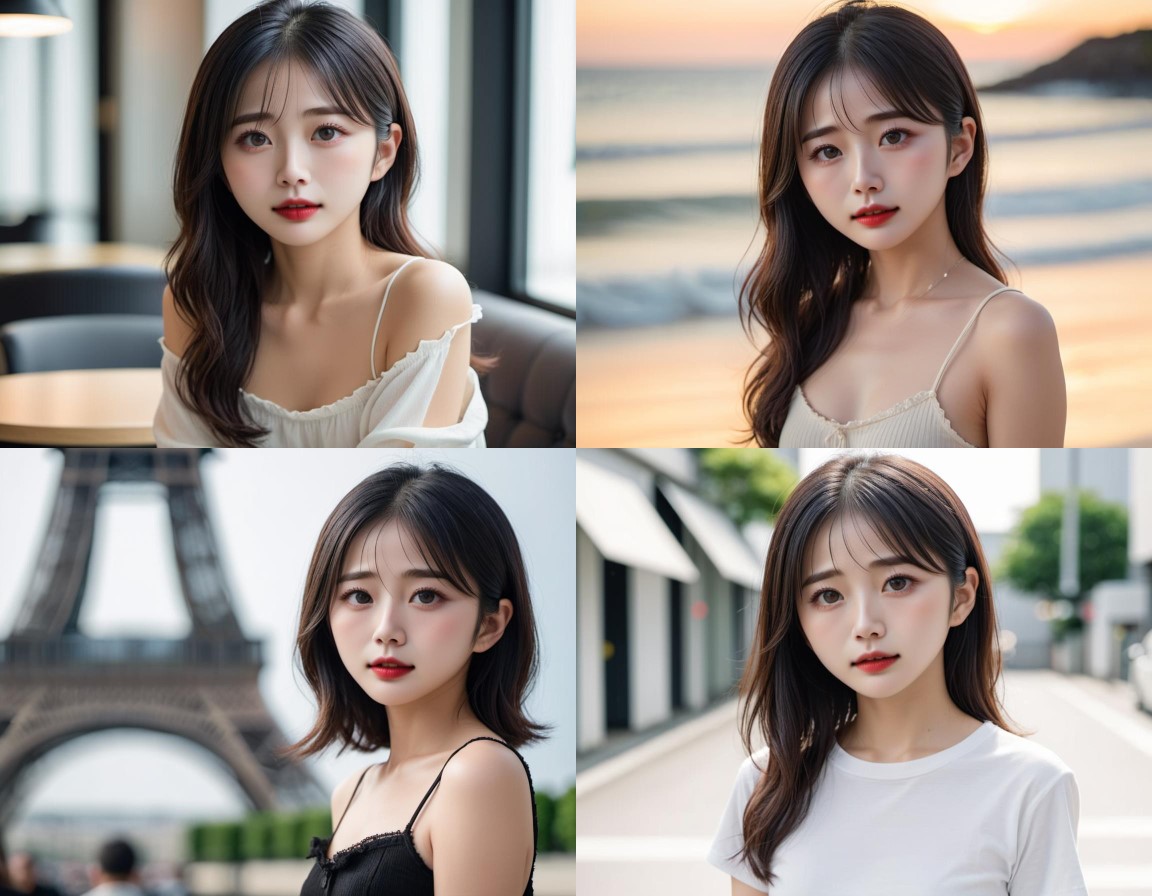
作成された4枚の画像に写る人物は同じ人に見えます。ただし、元画像の人と同一人物かと言えば疑問が残ります。
目的
今回は「IP-Adapter-FaceID-PlusV2」で同一人物の写真を大量に作成し、それをLoRA学習に使ってみようとする試みです。同一人物の写真を作成したいなら「IP-Adapter-FaceID-PlusV2」を使えば十分です。今回の目的は一人の人物の写真が複数枚用意できた時に、LoRAでどこまでその人物を再現できるかを試すことです。「IP-Adapter-FaceID-PlusV2」は学習用データを作るだけに使いました。
最近Pivotal Tuningというのを組み合わせたLoRAが発表されました。それを使ってみようと思います。
huggingface.co
Pythonスクリプト
以下のスクリプトで120枚の学習用データを作成しました。import os import cv2 from insightface.app import FaceAnalysis from insightface.utils import face_align import torch from diffusers import StableDiffusionXLPipeline, DPMSolverMultistepScheduler import itertools import argparse from ip_adapter.ip_adapter_faceid import IPAdapterFaceIDPlusXL parser = argparse.ArgumentParser() parser.add_argument( '--repeat', type=int, default=5, help="nuber of repeat", ) args = parser.parse_args() repeat = args.repeat image_size = 640 # image_size%112 or image_size%128 must be 0 app = FaceAnalysis(name="buffalo_l", providers=['CUDAExecutionProvider']) app.prepare(ctx_id=0, det_size=(640, 640)) image = cv2.imread("face.png") faces = app.get(image) faceid_embeds = torch.from_numpy(faces[0].normed_embedding).unsqueeze(0) face_image = face_align.norm_crop(image, landmark=faces[0].kps, image_size=image_size) base_model_path = "model/fudukiMix_v20" image_encoder_path = "CLIP-ViT-H-14-laion2B-s32B-b79K" ip_ckpt = "IP-Adapter-FaceID/ip-adapter-faceid-plusv2_sdxl.bin" device = "cuda" noise_scheduler = DPMSolverMultistepScheduler( num_train_timesteps=1000, beta_start=0.00085, beta_end=0.012, beta_schedule="scaled_linear", steps_offset=1, algorithm_type="sde-dpmsolver++", use_karras_sigmas=True, ) pipe = StableDiffusionXLPipeline.from_pretrained( base_model_path, scheduler=noise_scheduler, torch_dtype=torch.float16, variant="fp16" ) ip_model = IPAdapterFaceIDPlusXL(pipe, image_encoder_path, ip_ckpt, device) negative_prompt = "hand, hands, finger, cleavage, illustration, 3d, 2d, painting, cartoons, sketch, watercolor, monotone, kimono, crossed eyes, strabismus" model_name = os.path.basename(base_model_path) save_folder = f"face_dataset_for_train{model_name}" os.makedirs(save_folder, exist_ok=True) shot_list = ["face shot", "close-up shot"] angle_list = ["straight-on", "from side"] lighting_list = ["natural lighting", "cinematic lighting", "studio lighting"] for (shot, angle, lighting) in itertools.product(shot_list, angle_list, lighting_list): for i in range(repeat): prompt = f"japanese woman, wavy medium hair, {shot}, {angle}, {lighting}, best quality" images = ip_model.generate( prompt=prompt, negative_prompt=negative_prompt, face_image=face_image, faceid_embeds=faceid_embeds, shortcut=True, s_scale=1.0, num_samples=1, width=1024, height=1024, num_inference_steps=40, guidance_scale=7.5 ) save_fname = ''.join(f"{model_name}_{shot}_{angle}_{lighting}_{i}.png".split()) images[0].save(os.path.join(save_folder, save_fname))
学習
つづきはこちらに書きました。touch-sp.hatenablog.com
Python環境構築(2024年9月11日追記)
pip install torch==2.3.1+cu118 --index-url https://download.pytorch.org/whl/cu118 pip install diffusers[torch] pip install transformers einops peft pip install git+https://github.com/tencent-ailab/IP-Adapter.git pip install onnxruntime-gpu==1.18.1 insightface